АНАЛІЗ АЛГОРИТМІВ РАННЬОГО ВИЯВЛЕННЯ КІБЕРАТАК НА МЕРЕЖІ З ВИКОРИСТАННЯМ МАШИННОГО НАВЧАННЯ
DOI:
https://doi.org/10.58254/viti.6.2024.08.105Ключові слова:
машинне навчання, виявлення кібератак, розподілена атака на відмову в обслуговуванні, виявлення мережевих вторгнень, нейронні мережіАнотація
Захист критичної інфраструктури та національна безпека посилюються завдяки безпеці та надійності
мереж. В цих мережах циркулює різна інформація з різними грифами від відкритої до закритої. Наслідки
кібератак на ці мережі можуть, бути серйозними, включаючи шкоду репутації, фінансові втрати, операційні
перебої та витік даних. Традиційні методи безпеки, такі як брандмауери та антивірусне програмне
забезпечення, стають все менш ефективними проти сучасних і постійно мінливих кіберзагроз. Як наслідок,
потужні системи виявлення мережевих вторгнень (IDS) стали незамінними для проактивного виявлення та
пом’якшення наслідків кібератак.
Машинне навчання стало життєздатним методом для створення адаптивних засобів виявлення
вторгнень, які можуть виявляти нові та складні шаблони атак. Навчаючись на величезних маркованих наборах
даних мережевого трафіку, моделі ML можуть розуміти тонкі закономірності і диференціальні ознаки
нормальних і аномальних або зловмисних потоків трафіку. Це дозволяє виявляти можливі кіберзагрози та
вторгнення, які традиційні IDS на основі сигнатур не можуть виявити. Виділення дискримінаційних ознак і
навчання відповідних моделей класифікації з таких даних є непростим завданням.
У представленому дослідженні проведено аналіз ефективності алгоритмів ML для виявлення кібератак,
зокрема розподілених атак на відмову в обслуговуванні DDoS, у даних мережевого трафіку. У представленому
дослідженні система виявлення мережевих атак розроблена з використанням моделей ML і глибинного навчання
(DL) та експериментується на наборі даних CICIDS2017.
Основними завданнями дослідження є розроблення стратегії вилучення цінної інформації з необроблених
мережевих потоків; вивчення впливу підготовки даних на частоту хибних спрацьовувань; проведення
порівняльного аналізу моделей ML для ідентифікації кібератак.
Основною метою дослідження є забезпечення розуміння розробки надійної адаптивної системи виявлення
мережевих вторгнень з використанням підходів ML, які підвищують можливості кібербезпеки та захищають
від майбутніх кібератак
Посилання:
- Li Y., Liu Q. A comprehensive review study of cyber-attacks and cyber security; Emerging trends
and recent developments. Energy Reports. 2021. URL: https://doi.org/10.1016/j.egyr.2021.08.126 (date of
access: 03.05.2024).
2. George A. S., George A. H. & Baskar T. Digitally Immune Systems: Building Robust Defences in
the Age of Cyber Threats. Partners Universal International Innovation Journal. 2023. Vol. 1. № 4.
P. 155–172. DOI: 10.5281/zenodo.8274514.
3. Explainable Intrusion Detection for Cyber Defences in the Internet of Things: Opportunities and
Solutions / N. Moustafa et al. IEEE Communications Surveys & Tutorials. 2023. P. 1.
URL: https://doi.org/10.1109/comst.2023.3280465 (date of access: 03.05.2024).
4. A Review of Intrusion Detection Systems Using Machine and Deep Learning in Internet of Things:
Challenges, Solutions and Future Directions / J. Asharf et al. Electronics. 2020. Vol. 9, no. 7. P. 1177.
URL: https://doi.org/10.3390/electronics9071177 (date of access: 03.05.2024).
5. An Experimental Analysis of Attack Classification Using Machine Learning in IoT Networks /
A. Churcher et al. Sensors. 2021. Vol. 21, no. 2. P. 446. URL: https://doi.org/10.3390/s21020446 (date of
access: 03.05.2024).
6. Evaluating Deep Learning Based Network Intrusion Detection System in Adversarial Environment /
Y. Peng et al. 2019 IEEE 9th International Conference on Electronics Information and Emergency
Communication (ICEIEC), Beijing, China, 12–14 July 2019. 2019. URL:
https://doi.org/10.1109/iceiec.2019.8784514 (date of access: 03.05.2024).
7. Intrusion Detection and Prevention Systems: An Updated Review / N. A. Azeez et al. Data
Management, Analytics and Innovation. Singapore, 2019. P. 685–696. URL: https://doi.org/10.1007/978-981-
32-9949-8_48 (date of access: 03.05.2024).
8. A Survey of Intrusion Detection Systems Leveraging Host Data / R. A. Bridges et al. ACM Computing
Surveys. 2020. Vol. 52, no. 6. P. 1–35. URL: https://doi.org/10.1145/3344382 (date of access: 03.05.2024).
9. Parizad A., Hatziadoniu C. Cyber-Attack Detection Using Principal Component Analysis and Noisy
Clustering Algorithms: A Collaborative Machine Learning-Based Framework. IEEE Transactions on Smart
Grid. 2022. Vol. 13, no. 6. Р. 4848-4861. URL: https://doi.org/10.1109/tsg.2022.3176311 (date of access:
03.05.2024).
10. Eliyan L. F., Di Pietro R. DoS and DDoS attacks in Software Defined Networks: A survey of
existing solutions and research challenges. Future Generation Computer Systems. 2021. Vol. 122. P. 149–171.
URL: https://doi.org/10.1016/j.future.2021.03.011 (date of access: 03.05.2024).
11. Alghawazi M., Alghazzawi D., Alarifi S. Detection of SQL Injection Attack Using Machine
Learning Techniques: A Systematic Literature Review. Journal of Cybersecurity and Privacy. 2022. Vol. 2,
no. 4. P. 764–777. URL: https://doi.org/10.3390/jcp2040039 (date of access: 03.05.2024).
12. SSH and FTP brute-force Attacks Detection in Computer Networks: LSTM and Machine Learning
Approaches / M. D. Hossain et al. 2020 5th International Conference on Computer and Communication
Systems (ICCCS), Shanghai, China, 15–18 May 2020. 2020. URL: https://doi.org/10.1109/
icccs49078.2020.9118459 (date of access: 03.05.2024).
13. Thankappan M., Rifà-Pous H., Garrigues C. Multi-Channel Man-in-the-Middle Attacks Against
Protected Wi-Fi Networks: A State of the Art Review. Expert Systems with Applications. 2022. Vol. 210.
P. 118401. URL: https://doi.org/10.1016/j.eswa.2022.118401 (date of access: 03.05.2024).
14. Almulla K. Cyber-attack detection in network traffic using machine learning. 2022. URL:
https://repository.rit.edu/cgi/viewcontent.cgi?article=12453&context=theses (date of access: 03.05.2024).
15. Deep Learning Approach for Intelligent Intrusion Detection System / R. Vinayakumar et al. IEEE
Access. 2019. Vol. 7. P. 41525–41550. URL: https://doi.org/10.1109/access.2019.2895334 (date of access:
03.05.2024).
16. BAT: Deep Learning Methods on Network Intrusion Detection Using NSL-KDD Dataset / T. Su et
al. IEEE Access. 2020. Vol. 8. P. 29575–29585. URL: https://doi.org/10.1109/access.2020.2972627 (date of
access: 03.05.2024).
17. Ding Y., Zhai Y. Intrusion Detection System for NSL-KDD Dataset Using Convolutional Neural
Networks. CSAI '18: Proceedings of the 2018 2nd International Conference on Computer Science and
Artificial Intelligence, Shenzhen, China, 8–10 December 2018. New York, USA, ACM Press, 2018. P. 81–85.
URL: https://doi.org/10.1145/3297156.3297230 (date of access: 03.05.2024).
18. Meena G., Choudhary R. R. A review paper on IDS classification using KDD 99 and NSL KDD
dataset in WEKA. 2017 International Conference on Computer, Communications and Electronics
(Comptelix), Jaipur, India, 1–2 July 2017. 2017. URL: https://doi.org/10.1109/comptelix.2017.8004032 (date
of access: 03.05.2024).
19. Benchmarking datasets for Anomaly-based Network Intrusion Detection: KDD CUP 99
alternatives / A. Divekar et al. 2018 IEEE 3rd International Conference on Computing, Communication and
Security (ICCCS), Kathmandu, 25–27 October 2018. 2018. URL: https://doi.org/10.1109/
cccs.2018.8586840 (date of access: 03.05.2024).
20. A Deep Learning Approach for Network Intrusion Detection Based on NSL-KDD Dataset /
C. Zhang et al. 2019 IEEE 13th International Conference on Anti-counterfeiting, Security, and Identification
(ASID), Xiamen, China, 25–27 October 2019. IEEE. P. 41–45. URL: https://doi.org/10.1109/
icasid.2019.8925239 (date of access: 04.05.2024).
21. CICIDS2017. URL: https://www.researchgate.net/figure/Description-of-files-containingCICIDS2017-dataset_tbl1_329045441 (date of access: 04.05.202
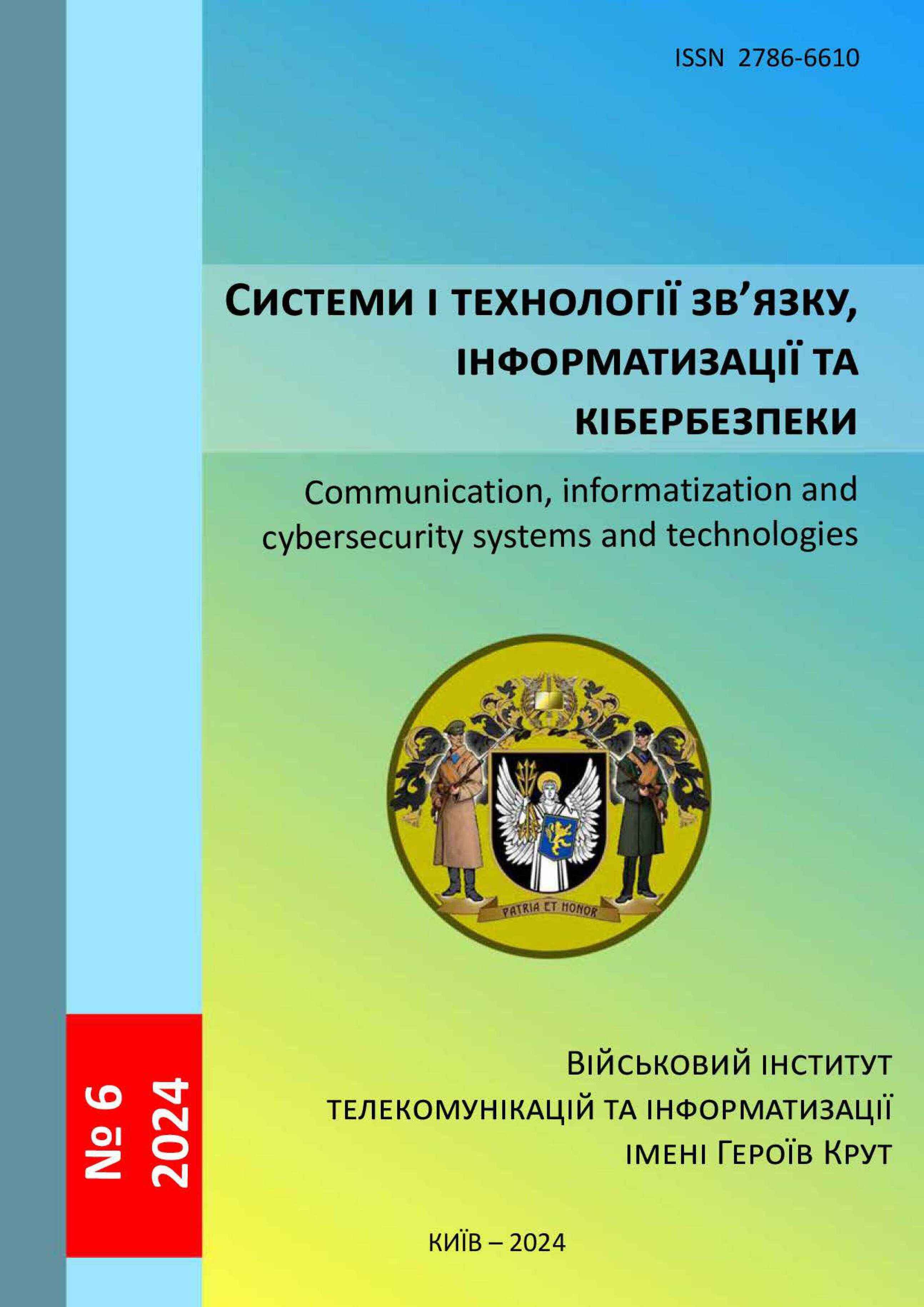