МОДЕЛІ ТА МЕТОДИ ВИЯВЛЕННЯ ВТОРГНЕНЬ У БЕЗПРОВОДОВИХ СЕНСОРНИХ МЕРЕЖАХ ТАКТИЧНОЇ ЛАНКИ УПРАВЛІННЯ ВІЙСЬКАМИ
DOI:
https://doi.org/10.58254/viti.6.2024.01.5Ключові слова:
безпроводові сенсорні мережі, виявлення вторгнень, управління безпекою, тактична ланка управління, нейронні мережі, виявлення аномаліїАнотація
Безпроводові сенсорні мережі є важливим елементом сучасних військових операцій, що забезпечують
моніторинг та передачу даних у реальному часі. Однак ці мережі вразливі до фізичних і кібератак через
обмеженість ресурсу, відсутність фізичного контролю над сенсорами та викликами, що пов’язані
з використання безпроводових каналів зв’язку. Метою статті є проведення порівняльного аналізу моделей та
методів виявлення вторгнень у безпроводових сенсорних мережах тактичної ланки управління військами.
Аналіз охоплює централізовані та децентралізовані підходи до управління безпекою з акцентом на моделі
виявлення, що базуються на сигнатурах, аномаліях та специфікаціях. Також у статті розкрито можливості
використання гібридних методів, що комбінують переваги вищезазначених підходів. Для порівняння
ефективності моделей використовувалися загальнодоступні набори даних (KDD, NSL-KDD, WSN-DS) та
синтетичні набори даних отриманих з використанням мережевих симуляторів. Результати показують, що
централізовані моделі більш ефективні для малих мереж, але створюють навантаження на базову станцію, що
може спричиняти затримки при виявленні атак. Децентралізовані моделі знижують навантаження та
підвищують швидкість реагування на атаки, проте також мають свої недоліки. У статті зазначено, що жоден
з існуючих методів не забезпечує повного захисту, тому комбінування підходів є найбільш ефективним рішенням.
Моделі та методи виявлення вторгнень на основі аномалій класифікуються залежно від їх функціональних
можливостей: на основі статистики, на основі інтелектуального аналізу даних, на основі машинного навчання
та на основі штучного інтелекту. Використання штучних нейронних мереж і машинного навчання значно
покращує точність виявлення аномалій, але такі системи вимагають великих обчислювальних ресурсів та
складні в налаштуванні.
Основний аналітичний висновок статті полягає в необхідності створення гібридної системи виявлення
вторгнень з використанням штучних нейронних мереж і машинного навчання, яка поєднує централізовані та
децентралізовані методи з урахуванням специфічних загроз для безпроводових сенсорних мереж тактичної
ланки управління військами.
Напрямом подальших досліджень слід вважати розроблення функціональної моделі системи виявлення
вторгнень для підсистеми безпеки у безпроводових сенсорних мережах тактичної ланки управління військами.
Посилання
- Міночкін А. І., Романюк В. А., Шаціло П. В. Виявлення атак в мобільних радіомереж. Збірник
наукових праць № 1. – Київ. ВІТІ НТУУ “КПІ”. – 2005. – С. 102-111. - Ghosal A., Halder S. A survey on energy efficient intrusion detection in wireless sensor
networks. Journal of Ambient Intelligence and Smart Environments. 2017. Vol. 9, no. 2. P. 239–261.
URL: https://doi.org/10.3233/ais-170426 (date of access: 07.10.2024). - Артюх С.Г., Жук О.В., Чернега В.М. Класифікація атак у безпроводових сенсорних мережах
тактичної ланки управління військами. Сучасні інформаційні технології у сфері безпеки та оборони.
2023.Т. 48. № 3, 2023. С.11-19. - On the Vital Areas of Intrusion Detection Systems in Wireless Sensor Networks / A. Abduvaliyev et
al. IEEE Communications Surveys & Tutorials. 2013. Vol. 15, no. 3. P. 1223–1237.
URL: https://doi.org/10.1109/surv.2012.121912.00006 (date of access: 08.10.2024).. - Alrajeh N. A., Khan S., Shams B. Intrusion Detection Systems in Wireless Sensor Networks:
A Review. International Journal of Distributed Sensor Networks. 2013. Vol. 9, no. 5. P. 167575.
URL: https://doi.org/10.1155/2013/167575 (date of access: 07.10.2024). - Osanaiye O. A., Alfa A. S., Hancke G. P. Denial of Service Defence for Resource Availability in
Wireless Sensor Networks. IEEE Access. 2018. Vol. 6. P. 6975–7004.
URL: https://doi.org/10.1109/access.2018.2793841 (date of access: 08.10.2024). - Alrajeh N. A., Lloret J. Intrusion Detection Systems Based on Artificial Intelligence Techniques in
Wireless Sensor Networks. International Journal of Distributed Sensor Networks. 2013. Vol. 9, no. 10.
P. 351047. URL: https://doi.org/10.1155/2013/351047 (date of access: 08.10.2024). - Intrusion Detection System Based on Evolving Rules for Wireless Sensor Networks / N. Lu et
al. Journal of Sensors. 2018. Vol. 2018. P. 1–8. URL: https://doi.org/10.1155/2018/5948146 (date of access:
08.10.2024). - A Partially Distributed Intrusion Detection System for Wireless Sensor Networks / E. Cho et
al. Sensors. 2013. Vol. 13, no. 12. P. 15863–15879. URL: https://doi.org/10.3390/s131215863 (date of access:
08.10.2024). - Centralized IDS Based on Misuse Detection for Cluster-Based Wireless Sensors Networks /
F. Hidoussi et al. Wireless Personal Communications. 2015. Vol. 85, no. 1. P. 207–224. - Hierarchical Abnormal-Node Detection Using Fuzzy Logic for ECA Rule-Based Wireless Sensor
Networks / N. Berjab et al. 2018 IEEE 23rd Pacific Rim International Symposium on Dependable Computing
(PRDC), Taipei, Taiwan, 4–7 December 2018. 2018. URL: https://doi.org/10.1109/prdc.2018.00051 (date of
access: 08.10.2024). - Ioannou C., Vassiliou V. An Intrusion Detection System for Constrained WSN and IoT Nodes Based
on Binary Logistic Regression. MSWIM '18: 21st ACM Int'l Conference on Modelling, Analysis and
Simulation of Wireless and Mobile Systems, Montreal QC Canada. New York, NY, USA, 2018.
URL: https://doi.org/10.1145/3242102.3242145 (date of access: 08.10.2024). - Osanaiye O. A., Alfa A. S., Hancke G. P. Denial of Service Defence for Resource Availability in
Wireless Sensor Networks. IEEE Access. 2018. Vol. 6. P. 6975–7004.
URL: https://doi.org/10.1109/access.2018.2793841 (date of access: 08.10.2024). - Intrusion detection model of wireless sensor networks based on game theory and an autoregressive
model / L. Han et al. Information Sciences. 2019. Vol. 476. P. 491–504.
URL: https://doi.org/10.1016/j.ins.2018.06.017 (date of access: 08.10.2024). - Detection and mitigation of sinkhole attacks in wireless sensor networks / H. Shafiei et al. Journal
of Computer and System Sciences. 2014. Vol. 80, no. 3. P. 644–653.
URL: https://doi.org/10.1016/j.jcss.2013.06.016 (date of access: 08.10.2024). - Ballarini P., Mokdad L., Monnet Q. Modeling tools for detecting DoS attacks in WSNs. Security
and Communication Networks. 2013. Vol. 6, no. 4. P. 420–436. - Kaur G., Singh M. Detection of black hole in Wireless Sensor Network based on Data Mining. 2014
5th International Conference- Confluence The Next Generation Information Technology Summit, Noida, India,
25–26 September 2014. 2014. URL: https://doi.org/10.1109/confluence.2014.6949343 (date of access:
08.10.2024). - Hybrid Anomaly Detection by Using Clustering for Wireless Sensor Network / B. Ahmad et
al. Wireless Personal Communications. 2018. Vol. 106, no. 4. P. 1841–1853.
URL: https://doi.org/10.1007/s11277-018-5721-6 (date of access: 08.10.2024). - Applying Data Mining Techniques to Intrusion Detection in Wireless Sensor Networks /
L. Coppolino et al. 2013 Eighth International Conference on P2P, Parallel, Grid, Cloud and Internet
Computing (3PGCIC), COMPIEGNE, France, 28–30 October 2013. 2013. - A New Intrusion Detection System Based on KNN Classification Algorithm in Wireless Sensor
Network / W. Li et al. Journal of Electrical and Computer Engineering. 2014. Vol. 2014. P. 1–8.
URL: https://doi.org/10.1155/2014/240217 (date of access: 08.10.2024). - Garofalo A., Di Sarno C., Formicola V. Enhancing Intrusion Detection in Wireless Sensor Networks
through Decision Trees. Lecture Notes in Computer Science. Berlin, Heidelberg, 2013. P. 1–15.
URL: https://doi.org/10.1007/978-3-642-38789-0_1 (date of access: 08.10.2024).. - Cooperative game theoretic approach using fuzzy Q-learning for detecting and preventing intrusions
in wireless sensor networks / S. Shamshirband et al. Engineering Applications of Artificial Intelligence. 2014.
Vol. 32. P. 228–241. URL: https://doi.org/10.1016/j.engappai.2014.02.001 (date of access: 08.10.2024). - Wang H., Wen Y., Zhao D. Identifying localization attacks in wireless sensor networks using deep
learning. Journal of Intelligent & Fuzzy Systems. 2018. Vol. 35, no. 2. P. 1339–1351.
URL: https://doi.org/10.3233/jifs-169677 (date of access: 08.10.2024). - A Lightweight Intrusion Detection Method Based on Fuzzy Clustering Algorithm for Wireless
Sensor Networks / H. Qu et al. Advances in Fuzzy Systems. 2018. Vol. 2018. P. 1–12.
URL: https://doi.org/10.1155/2018/4071851 (date of access: 08.10.2024). - Wireless Sensor Networks Intrusion Detection Based on SMOTE and the Random Forest
Algorithm / X. Tan et al. Sensors. 2019. Vol. 19, no. 1. P. 203. URL: https://doi.org/10.3390/s19010203 (date
of access: 08.10.2024). - Mansouri A., Majidi B., Shamisa A. Metaheuristic neural networks for anomaly recognition in
industrial sensor networks with packet latency and jitter for smart infrastructures. International Journal of
Computers and Applications. 2018. P. 1–10. URL: https://doi.org/10.1080/1206212x.2018.1533613 (date of
access: 08.10.2024). - Bitam S., Zeadally S., Mellouk A. Bio-inspired cybersecurity for wireless sensor networks. IEEE
Communications Magazine. 2016. Vol. 54, no. 6. P. 68–74. - N. Nithiyanandam, P. Latha Parthiban, B. Rajalingam. Effectively Suppress the Attack of Sinkhole
in Wireless Sensor Network using Enhanced Particle Swarm Optimization Technique. International Journal
of Pure and Applied Mathematics, Vol. 118, No. 9, pp. 313-329, 2018. - An Integrated Intrusion Detection Model of Cluster-Based Wireless Sensor Network / X. Sun et
al. PLOS ONE. 2015. Vol. 10, no. 10. P. e0139513. URL: https://doi.org/10.1371/journal.pone.0139513 (date
of access: 08.10.2024). - A Hybrid Spectral Clustering and Deep Neural Network Ensemble Algorithm for Intrusion
Detection in Sensor Networks / T. Ma et al. Sensors. 2016. Vol. 16, no. 10. P. 1701.
URL: https://doi.org/10.3390/s16101701 (date of access: 08.10.2024). - A Hybrid Spectral Clustering and Deep Neural Network Ensemble Algorithm for Intrusion
Detection in Sensor Networks / T. Ma et al. Sensors. 2016. Vol. 16, no. 10. P. 1701.
URL: https://doi.org/10.3390/s16101701 (date of access: 08.10.2024). - Sedjelmaci H., Senouci S. M., Feham M. An efficient intrusion detection framework in clusterbased wireless sensor networks. Security and Communication Networks. 2013. Vol. 6, no. 10. P. 1211–1224.
URL: https://doi.org/10.1002/sec.687 (date of access: 08.10.2024). - Hybrid Intrusion Detection System for enhancing the security of a cluster-based Wireless Sensor
Network / K. Q. Yan et al. 2010 3rd IEEE International Conference on Computer Science and Information
Technology (ICCSIT 2010), Chengdu, China, 9–11 July 2010. 2010. URL: https://doi.org/10.1109/
iccsit.2010.5563886 (date of access: 08.10.2024). - Subba B., Biswas S., Karmakar S. A Game Theory Based Multi Layered Intrusion Detection
Framework for Wireless Sensor Networks. International Journal of Wireless Information Networks. 2018.
Vol. 25, no. 4. P. 399–421. URL: https://doi.org/10.1007/s10776-018-0403-6 (date of access: 08.10.2024). - Otoum S., Kantarci B., Mouftah H. T. Detection of Known and Unknown Intrusive Sensor Behavior
in Critical Applications. IEEE Sensors Letters. 2017. Vol. 1, no. 5. P. 1–4. URL: https://doi.org/10.1109/
lsens.2017.2752719 (date of access: 08.10.2024).
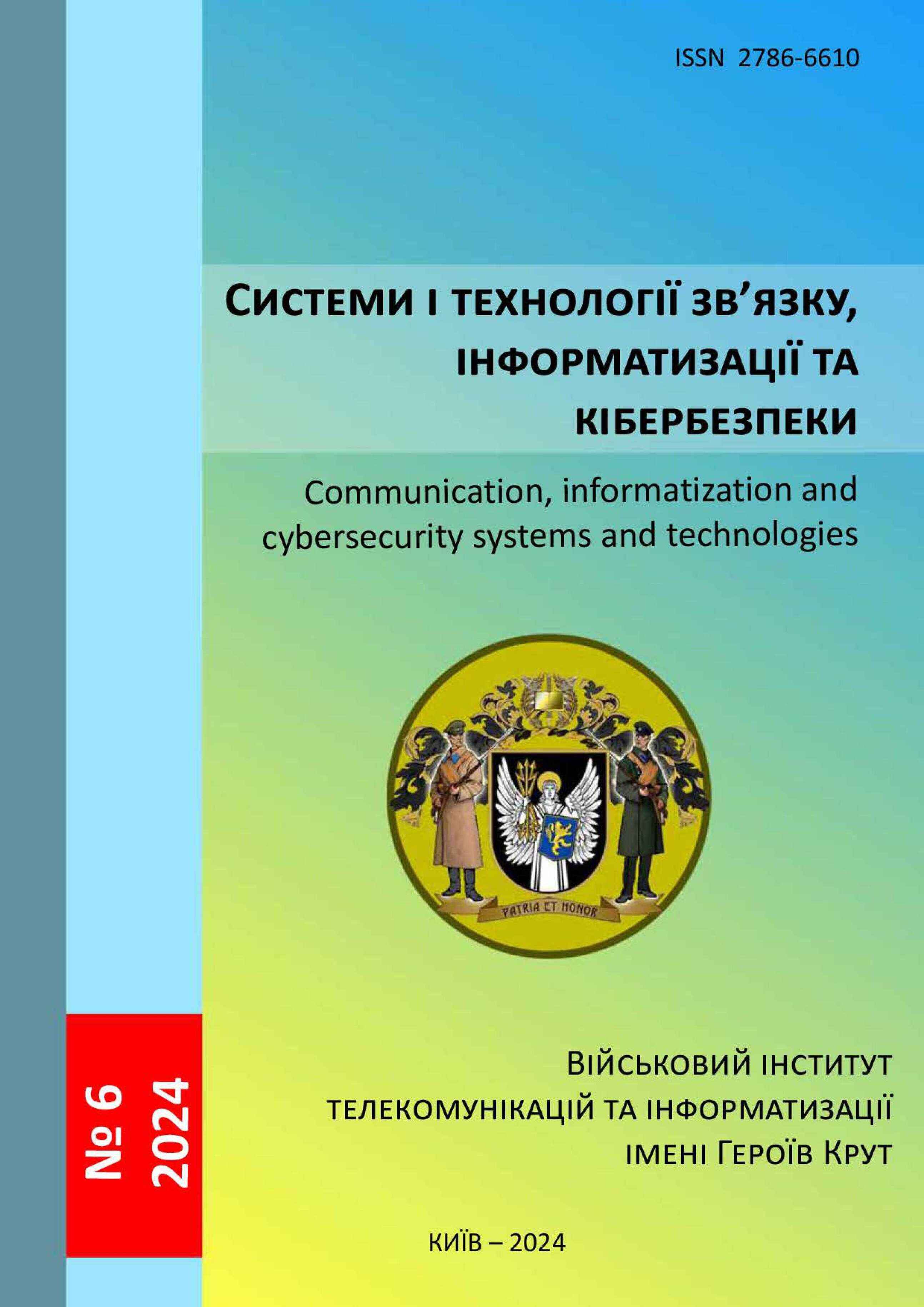